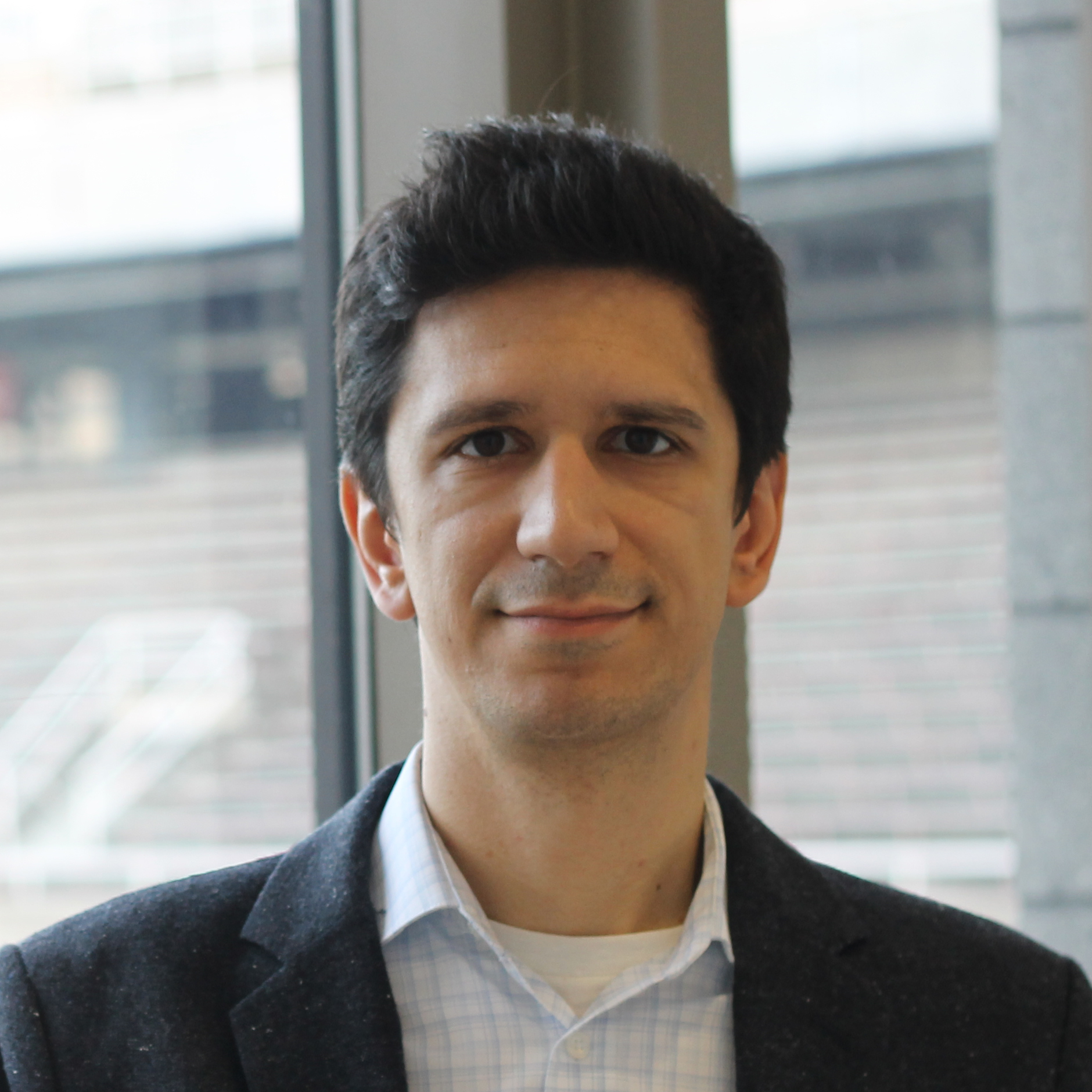
Bratislav Misic
Associate Professor & Canada Research Chair
Montreal Neurological Institute, McGill University
Learning in connectome-based artificial neural networks
The connection patterns of neural circuits in the brain form a complex network. Collective signalling within the network manifests
as patterned neural activity and is thought to support human cognition and adaptive behaviour. Recent technological advances
permit macroscale reconstructions of biological brain networks. These maps, termed connectomes, display multiple non-random
architectural features, including heavy-tailed degree distributions, segregated communities and a densely interconnected core. Yet,
how computation and functional specialization emerge from network architecture remains unknown. Here we reconstruct human
brain connectomes using in vivo diffusion-weighted imaging and use reservoir computing to implement connectomes as artificial
neural networks. We then train these neuromorphic networks to learn a memory-encoding task. We show that biologically realistic
neural architectures perform best when they display critical dynamics. We find that performance is driven by network topology and
that the modular organization of intrinsic networks is computationally relevant. We observe a prominent interaction between network
structure and dynamics throughout, such that the same underlying architecture can support a wide range of memory capacity
values as well as different functions (encoding or decoding), depending on the dynamical regime the network is in. This work opens
new opportunities to discover how the network organization of the brain optimizes cognitive capacity.